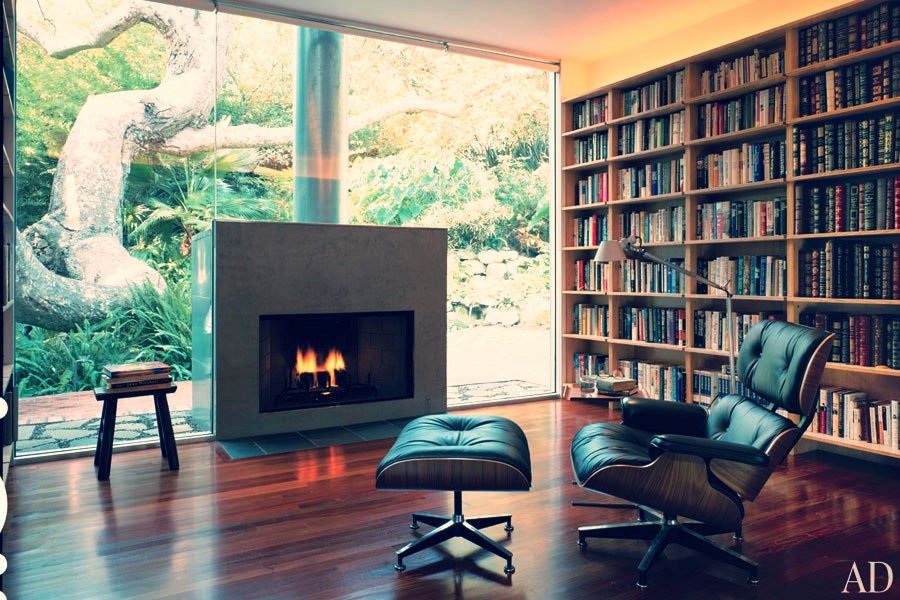
A COLLECTION OF THOUGHTS
Thoughts
&
Musings
6 Indicators You Need a Data Science Team
In our digital economy every organization generates a sizable amount of data. There is real value in understanding and acting on insights and solutions that lay within this data. To be successful at gathering insights from data an organization needs a team of experts with various skill sets to complement each other and work collectively towards a common objective of getting value from the organization's data.
All organizations are not equal. The volume and variety of data differs, therefore, each organization has its unique challenges. The types of challenges faced dictate the type of experts that you need to consider bringing on board.
In our digital economy every organization generates a sizable amount of data. There is real value in understanding and acting on insights and solutions that lay within this data. To be successful at gathering insights from data an organization needs a team of experts with various skill sets to complement each other and work collectively towards a common objective of getting value from the organization's data.
All organizations are not equal. The volume and variety of data differs, therefore, each organization has its unique challenges. The types of challenges faced dictate the type of experts that you need to consider bringing on board.
If you find that your organization is facing these challenges you may need to hire a dc Analyst data science team to help simplify your needs:
Receiving multiple data using various sources and team members
Your IT or supervisory team is creating company performance reports
Your marketing and sales teams is in need of statistical analysis for campaigns
Your company is struggling to wrangle and organize your ever growing database
In this article we will discuss the different challenges organizations face and data analysts experts that often help organizations overcome those challenges.
Multiple Data Sources
At the most basic level data analysis is done using spreadsheets and various reports provided by varying team members. This approach has several shortcomings. First there is no standardized way of importing the data and applying necessary transformations on the data according to the organization’s business rules and objectives.
With every person doing data analysis on areas they feel is important the key performance indicators are difficult to identify. Secondly, due to the first shortcoming different people doing analysis on the same business processes are likely to arrive at different conclusions. This confusion wastes valuable time as it is lost investigating where differences come from instead of using data to collectively improve business operations. Thirdly, creation of multiple copies of data from various sources is to reconcile in the process of investigating and wrangling data.
When such problems arise within an organization it is time to bring in an data analyst expert who is skilled at integrating multiple data sources into a single repository using business rules. The single repository then becomes the common data source that is relied upon for information across the organization for data analysis and reporting.
Data analyst with the ability to gather, organize, and present data are often referred as data architects, data engineers, or ETL developers. These experts have an important role of ensuring data quality and consistency.
Relying on IT to Create Reports
When your organization constantly relies on your IT team to create business reports an unacceptable load is placed on the IT team. Valuable time is also lost waiting for reports to be gathered and presented. IT teams have a distinct role within your organization that involves the maintenance and planning for your technology needs.
When reports are created by your IT team they may fall short of what is required by your business team. To avoid a lack of information consider asking a business intelligence (BI) developer to handle some of your data processing needs.
A BI developer acts as a liaison between your business team and your reporting needs. They are uniquely experienced in helping you understand their reporting needs. BI developers create reports and dashboards that can be used by your business team to meet their needs without relying on IT. The reports can also be scheduled to run at specified intervals of time and automatically sent to those who need them. This is referred to as self-service reporting.
Need for Statistical Data Analysis
Marketing. If your organization needs statistical analysis on market research data, experimental data, or data stored in a warehouse a data analyst should join your team. Data analysts help design surveys and systems that can help you understand your customers. Information data analysts can draw inferences from data to help you understand your customer preferences and buying habits. They also prepare reports that effectively communicate results of statistical analyses in simple and easy-to-understand presentations.
Manufacturing. Data analysts support engineers and scientists with information they gain from their investigations. They interpret data to enable scientific and manufacturing efforts. For example, a data analyst will help an engineer design an experiment to identify optimal manufacturing conditions. Another example is a data analyst partnering with a medical investigator to conduct a clinical trial of a new drug and obtaining market approval.
In addition, data analyst help organizations implement data driven quality improvement programs like 6 sigma. Armed with such information your business is able to optimize business processes. In many cases, data analysts can also train team members on how to analyze and interpret data.
Unable to Cope with Data Growth
In every organization there are data growth projections and measures devised to cope with growth in data volume. When the systems in place can no longer handle new data volumes it is time to bring in experts skilled in application of big data technologies. Signs of inability to handle growth in data volumes include reports taking too long to run, spending a lot of time tuning queries, and trying to split analytical databases.
When existing systems cannot handle new types of data it is important to implement an alternative system to ensure your data is accurate and usable. Data analysts are able to leverage technologies such as Hadoop and NoSQL databases to ensure analytical operations continue.
Predictive Analytics Are Required
If your organization realizes the need for deeper analytics beyond reporting than bringing in a data science expert is the recommended next step. A data scientist is able to pose the right questions that have business value, use data to get answers, and effectively communicate to decision makers.
In many cases organizations can use predictive insights to capture relationships that exist within their data. Examples of such needs include: predicting buying behavior from demographic data and purchase history, segmenting customers into different groups, and recommending products based on the findings. Data scientists apply predictive models on the data infrastructure created by a data engineers to gain insight from the data and communicate such insights to decision makers.
Integrating Analytics with Products
If your organization needs analytic insights to be integrated into a product then your software developer who will work closely with a data scientist. For example, a data scientist develops a predictive model that recommends products that were bought by similar customers. The data scientist and the software developer will work closely to sure the recommendation engine is properly implemented in the shopping cart. Another example of a software engineer and a data scientist working together is when a credit company uses a predictive model to score clients. Or an application for credit managers is developed to help them quickly score customers.
Determining if you need a data analyst or data science team requires a practical look at the way your organization is operating. Pay attention to these high level indicators as well as consult a dc Analyst team member to learn more about how your company can benefit from gathering, organizing, and interpreting your data.
How to Present Data and Findings
Modern business operations generate a variety of data from processes such as sales, customer relationships, human resource management, and product ordering. These multiple data sources are brought into a single repository. Often data analyst create reports for decision makers to aid in decision making and organizational planning.
Business intelligence (BI) tools are used to identify insights from data repositories. These BI tools connect to different data sources and enable data analysts to equip decision makers with relevant insights from the data. BI tools offer features that are useful for reporting, querying data, online analytical processing (OLAP), and data mining. In this article we will discuss each BI activity and how they are supported in Tableau, QlikView, and Excel. Lastly, we will look at how PowerPoint can be used to prepare presentations to effectively communicate findings.
Modern business operations generate a variety of data from processes such as sales, customer relationships, human resource management, and product ordering. These multiple data sources are brought into a single repository. Often data analyst create reports for decision makers to aide in decision making and organizational planning.
Business intelligence (BI) tools are used to identify insights from data repositories. These BI tools connect to different data sources and enable data analysts to equip decision makers with relevant insights from the data. BI tools offer features that are useful for reporting, querying data, online analytical processing (OLAP), and data mining. In this article we will discuss each BI activity and how they are supported in Tableau, QlikView, and Excel. Lastly, we will look at how PowerPoint can be used to prepare presentations to effectively communicate findings.
Reporting and Querying
Business reports are pre-defined ways of understanding your data. These reports are delivered on a regular schedule, such as weekly, or upon request. Reports are predefined. Using data querying you are able to select the type of data you would like to see. Reports and queries are easily visualized using cross tabulations and charts. In a cross tabulation the information is presented in rows and columns. Other ways to present data include charts such as pie, bar, and histogram. These tools help you understand your data and key performance indicators.
One of the most important parts of data are key performance indicators. To present a set of key performance indicators (KPIs) that provide a high level overview of your business dashboards are used. Just like in a car dashboard you are able to view all aspects of your business on a single location. A dashboard can contain business metrics displayed in charts and graphs, maps, KPIs, RSS feeds, and any other content that is viewable on the web. These dashboards can be updated daily, in real time, or via a monthly sales summary report.
OLAP
OLAP is a technique for exploring data interactively such as when you observe something interesting in your data you can immediately continue exploring the data to get answers. Using OLAP you are able to see data from multidimensional perspectives and drill up or down to view less or more details. Using OLAP a sales analyst can view sales data from one state for the month of April and the compare sales of the same product in August in comparison to other products that were sold.
Data Mining
Data mining is a collection of techniques that is used to understand data stored in databases. With data mining you are able to identify data anomalies, patterns, and relationships that exist in your data. Armed with this information you are able to grow revenue, reduce costs, identify fraud, improve customer relationship, and reduce risk exposure. With data mining we are also able to accomplish useful tasks such as predicting customers who are likely to purchase a product, transactions that are likely to be fraudulent, and possible cyber security breaches. By taking action on such insights your data analyst will provide recommendations on how to improve your business outcomes.
Tableau
Tableau is a BI tool available for use on a desktop, mobile device, a server, or as a hosted solution. With its availability on these various platforms it is an excellent tool for understanding and navigating data. With Tableau you are able to source data from files, relational databases, and Hadoop. Tableau has an excellent support for data reporting and visualization.
With Tableau you are not limited to reporting on raw data as you can perform calculations and use calculated fields in your reports. Simple and advanced data visualization features like waterfall diagrams, box plots, bump plots and histograms among others are supported.
Dashboards are very well supported in Tableau. For complex statistical functions not supported within Tableau you can easily use R. Integration of R and Tableau means you are easily able to implement data mining that enables you to understand hidden patterns in your data.
QlikView
With QlikView you are able to import data from different sources including files, the web, databases, and custom data sources. QlikView can be broadly divided into two parts which are the front end and the backend. The front end is a web browser based interface that enables users to explore and interact with data. The frontend has a QlikView server for viewing already created business reports which makes it easy to provide versatile reports. The back end is made up of QlikView desktop and QlikView publisher.
The desktop is used to create report templates which are viewed using a web browser. The publisher is used to distribute reports by controlling users who are allowed to view content and the type of content they can view. With QlikView you can analyze data using cross tabulations, charts, and statistical tests. Reporting, querying, and dashboards are very well supported.
Excel
Business Intelligence capabilities in Excel are almost at par with those of specialized tools because of features provided by Power BI. These features or add ons include Power Pivot, Power View, Power Map and Power Query. With Power Pivot you are able to import data from other spreadsheets, files, and databases. After importing data you can do analysis. Power View is the dashboard creation solution in Excel.
After creating a Power Pivot connection to data you are able to analyze your data using interactive reports and views. The charts, maps and tables created with Power View are interactive therefore you can drill down and segment to better understand your data. Once you have created dashboards you can present them within Power View or use a specialized presentation tool like PowerPoint. To visualize geographic information you use can use Power Map.
With Power Map supports OLAP in Excel and is very advanced. You are able to connect to Microsoft and non-Microsoft OLAP data sources as long as they offer OLEDB for OLAP support. Keep in mind that analysis of OLAP data is only possible using a Pivot Table or Pivot Chart.
PowerPoint
PowerPoint provides all features necessary to create presentations that effectively communicate insights from your data. It is most commonly used by data analyst. PowerPoint being a Microsoft product integrates very well with BI features in Excel. Dashboards created with PowerPivot are easily exported to PowerPoint. QlikView offers a plugin to help with the creation of PowerPoint presentations of charts and dashboards. Tableau offers features to export your visualizations as pdf files and also create PowerPoint presentations.
Presenting your data is essential for understanding your data. Data analysts must present recommendations and insights gathered from data to do a variety of things such as improve operations or project next quarter’s sales.
At dc Analyst we understand what it takes to present your findings and data in a way that makes sense. Our analysts can help you learn the basics of presenting data and findings to help you communicate your findings with your entire team.
How to Analyze Data
After your team and data analyst have finished setting your objectives and gathering data you need to analyze your data to meet your objectives. When analyzing data you can use descriptive, visual, inferential, or modeling techniques. In this article we discuss various data analysis techniques and tools to use in analyzing your data.
Summarizing Data Using Descriptive Statistics
Descriptive statistics help you summarize and understand your data. There are different techniques for summarizing your data depending on if your data is categorical or continuous. Categorical data refers to observations that fall into distinct categories for example male or female. Continuous data refers to observations that do not have any distinct categories such as weight.
After your team and data analyst have finished setting your objectives and gathering data you need to analyze your data to meet your objectives. When analyzing data you can use descriptive, visual, inferential, or modeling techniques. In this article we discuss various data analysis techniques and tools to use in analyzing your data.
Summarizing Data Using Descriptive Statistics
Descriptive statistics help you summarize and understand your data. There are different techniques for summarizing your data depending on if your data is categorical or continuous. Categorical data refers to observations that fall into distinct categories for example male or female. Continuous data refers to observations that do not have any distinct categories such as weight.
When your data is categorical the most useful descriptive technique to use is count. You count the number of observations that occur in each category. For example, when you have one variable such as gender you count the number of people who are male and those who are female. When you would like to know the number of people in each category as a proportion of the total you use a percentage. In the gender example we can calculate the percentage of those who are male and the percentage of those who are female.
As you summarize categorical data you are not limited to one variable. To summarize into categorical variables we use a cross tabulation. In a cross tabulation one variable forms the rows and the other variable forms categories. We then count the number of observations that fall in each category. If in our example we also have an education variable we would be interested in knowing the education levels of males and females. These education variables could be defined categories: no education, primary, secondary, college and university.
For continuous variables there are descriptive measures that tell us how our observations cluster around a single value and those that tell us how our observations are spread. The mean and the median are two common measures that are used to summarize data. The mean is an appropriate measure when we have observations almost falling on either side. The median is an appropriate summary when we have most observations falling on one side such as our observations are skewed.
If we collect observations on weight of adult patients we can use the mean to get the typical weight of a patient. If we collect observations on salaries we will have a few people earning much more than others, in that case the median would be a better summary.
The minimum, the maximum, the range, and the standard deviation tell us how observations are spread. The minimum tells us the lowest observation, the maximum tells us the highest observation, and the range gives us the difference between the lowest and the highest observation in our data. The variance and the standard deviation tell us how a mean value varies.
The confidence interval is calculated from the standard deviation and it gives us the upper and lower bounds of a mean value. When you have two continuous variables a correlation coefficient helps you understand the strength and direction of relationship.
A negative coefficient shows you when one variable increases the other variable decreases. A positive coefficient shows you when one variable increases the other variable decreases. A correlation value close to zero shows you there is weak or no relationship. A value of 0.5 shows moderate strength while a value close to 1 shows you there is a strong relationship.
Visualizing Data With Graphs
There are different tools for visualizing categorical and continuous data. To visualize categorical data you use a pie chart or a bar chart. A pie chart divides a circular shape into angular portions that enable you to see the count or percentage of observations that are in each category. A pie chart can only be used to visualize one categorical variable. A bar chart helps you visualize categorical data using vertical or horizontal bars that show you the count or percentage of observations in each category.
You can add the count or percentage of each category on the bars for easy comparison. Bars that are taller than the others show more observations in those categories. A bar chart can be used to summarize one or two categorical variables.
To visualize continuous observations you can use a histogram, a box plot, a scatter plot or a line plot. A histogram uses bars similar to a bar chart to visualize continuous observations. The key difference is that bars in a bar plot are for a single category while bars in a histogram show a range of values. A box plot summarizes data using a box and whiskers. The whiskers on both ends of the box plot show you the minimum and maximum observations in your data. Observations that lie beyond the whiskers are outliers.
The box shows you where half of your observations lie and within the box there is a line that shows you where the median lies. The histogram and box plot are useful for visualizing the distribution of your observations. The scatterplot helps you visualize the relationship between two continuous variables. It helps you visualize the direction and strength numerically shown by a correlation coefficient.
Making Inferences From Data
The techniques we have discussed so far help you summarize your data. To test hypotheses about your data you use inferential techniques. There are different techniques for continuous and categorical variables.
A Chi-square test helps you test if there is any relationship between categorical variables. For example, in summarizing categorical data example we can use a Chi-square to test if education levels of men and women differ. For continuous variables we are mostly interested in the mean, where we can use T tests or analysis of variance (ANOVA).
There are three variants of the T test that help us test if the mean of one variable differs from a target mean, if the means of two variables differ and if the mean of one variable differs at two different time points. ANOVA extends T tests by helping us test if more than two means are different.
To help support the process of data analysis your data analysts will use both commercial and open source tools have been developed. Popular commercial data analysis tools include IBM SPSS, SAS, Stata, Excel, and Minitab. These tools provide a graphical user interface and a programming language for data analysis. R is a popular open source tool that is used to analyze data by writing programs. All of the tools and techniques we have mentioned support all the data analysis techniques we have discussed.